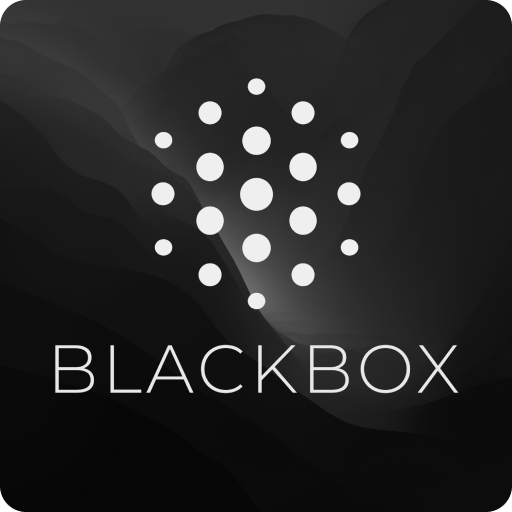
Artificial intelligence (AI) is revolutionizing the world, but how it works can often feel like a mystery. This is especially true for complex AI systems known as blackbox AI. These powerful tools deliver impressive results, but their inner workings remain shrouded in secrecy. In this blog post, we’ll delve into the world of black box AI, exploring its applications, benefits, drawbacks, and the ongoing quest for transparency.
What is BlackBox AI?
Black box AI refers to a type of machine learning model where the internal decision-making process is opaque. We feed the model data and get an output (prediction, classification, etc.), but the intricate calculations and reasoning behind that output remain hidden. Imagine a black box: you put something in (data) and get something out (result), but you can’t see what happens inside.
How Does BlackBox AI Work?
Black box AI models typically rely on complex algorithms like deep neural networks. These algorithms are trained on massive datasets, allowing them to identify patterns and relationships within the data. Over time, the model learns to make accurate predictions or classifications without being explicitly programmed with the underlying rules.
The Power of Black Box AI: Unleashing Potential
Despite the lack of transparency, black box AI offers significant advantages:
- High Accuracy: Black box models can achieve remarkable accuracy in tasks like image recognition, natural language processing, and fraud detection.
- Scalability: They can handle vast amounts of data, making them ideal for big data applications.
- Efficiency: Black box models can learn and adapt without the need for extensive human intervention, saving time and resources.
Black box AI is already transforming numerous industries:
- Healthcare: Diagnosing diseases, analyzing medical images, and predicting patient outcomes.
- Finance: Detecting fraud, managing risk, and personalizing financial products.
- Retail: Recommending products, optimizing pricing strategies, and understanding customer behavior.
- Self-Driving Cars: Making critical decisions in real-time based on sensor data and environmental conditions.
SAP Conversational AI | Leveraging AI and Machine Learning in SAP | How would AI look like in 2030
The Black Box Dilemma: A Tradeoff Between Performance and Explainability
While black box AI is powerful, its lack of transparency raises concerns:
- Bias and Fairness: If the training data is biased, the model can perpetuate those biases in its outputs.
- Debugging and Improvement: It can be difficult to pinpoint why a model makes a particular decision, hindering troubleshooting and improvement.
- Accountability: In critical applications like loan approvals or criminal justice, it’s crucial to understand how decisions are made.
Opening the Black Box: The Quest for Explainable AI
Researchers are actively developing techniques for explainable AI (XAI), aimed at making black box models more transparent. Here are some approaches:
- Feature Importance: Identifying which data points have the most significant influence on the model’s output.
- Saliency Maps: Visualizing how the model interprets different parts of an input (e.g., an image).
- Counterfactual Reasoning: Understanding what changes in the input would lead the model to make a different prediction.
The Future of Black Box AI: Striking a Balance
Black box AI offers immense potential, but it’s crucial to address the transparency concerns. As XAI techniques continue to develop, we can expect a future where powerful AI models are paired with explainability tools, allowing for responsible and trustworthy applications.
This blog post has just scratched the surface of black box AI. If you’re interested in learning more, here are some suggested resources: